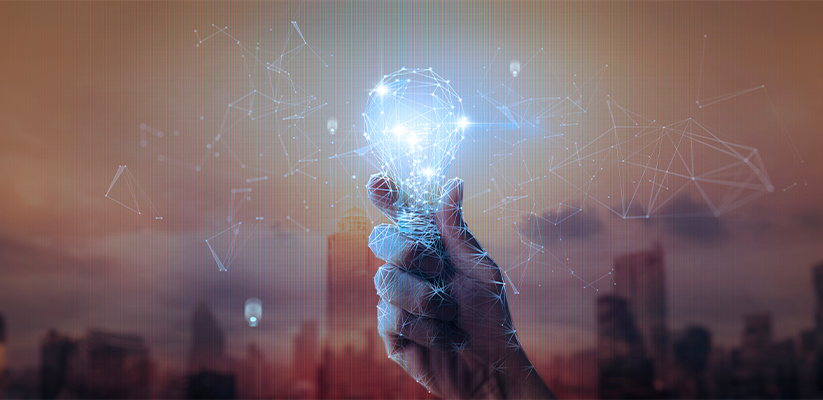
Organizations are constantly striving to harness the power of their data assets to make informed decisions and gain a competitive edge. This is why data mesh has emerged as a revolutionary approach to managing and scaling data infrastructure. Data mesh represents a paradigm shift, as it emphasizes decentralization, domain-oriented data ownership, and a collaborative approach to data management.
It follows four main principles:
- Domain oriented, decentralized data ownership: Each domain is in charge of managing and exposing its own data to the organization.
- Data as a Product: A data product is a dataset from a specific domain. Data Products should be easily discoverable, understandable, and accessible to the rest of the organization.
- Self-service data infrastructure as-a-platform, to avoid complexity and duplication of efforts across domains. This also enables domains to build, deploy, publish, and manage data products in a self-serve manner.
- Federated computational data governance ensures interoperability between domains through common semantics and conventions for shared entities. If necessary, global security and governance policies can be applied commonly to all domains.
Implementing a data mesh can be complex, but with the right tools, the process can be significantly streamlined.
Challenges of Data Mesh
Implementing a decentralized strategy can bring about transformative changes in how an organization manages and leverages its data. However, like any major strategy shift, it can present a few challenges that must be overcome to ensure a successful implementation.
- Mindset Shift: Transitioning from a traditional centralized data approach to a decentralized data mesh requires a fundamental shift in mindset. It involves redefining data ownership, sharing, and collaboration.
- Domain Ownership: Assigning data ownership to individual domain teams sounds straightforward in theory, but it can be complex in practice. Defining clear ownership, responsibilities, and accountability for data domains requires careful planning and communication.
- Data Governance: A decentralized approach can lead to challenges in maintaining consistent data quality, security, and compliance. Establishing governance frameworks that allow for autonomy while ensuring data integrity across domains is a delicate balance.
- Data Discovery: If numerous datasets are distributed across different domains, it can be challenging to discover relevant datasets and understand their qualities, context, and availability. It therefore becomes critical to implement effective data cataloging and discovery mechanisms.
- Data Sharing and Collaboration: For a successful data mesh implementation, it is essential to promote collaboration between domain teams so that they are open to sharing and consuming data products. Overcoming siloed data practices and fostering a culture of data sharing requires clear incentives and communication.
- Technical Infrastructure: The underlying technical infrastructure to support a decentralized architecture like data mesh should ensure scalability and good performance.
- Skillset Requirements: A mix of skills across domains, including data engineering, data science, and domain-specific expertise, are necessary for a successful data mesh implementation. It can be a challenge to ensure that team members possess the required skills and to facilitate skill development.
- Data Security and Privacy: With data distributed across domains, maintaining data security and privacy becomes more complex. Implementing robust data access controls, policies, and compliance measures, while enabling data sharing, requires careful planning.
This is why a data management platform that bridges the gap between complex technical processes and the practical needs of business users would be necessary.
Data Democratization
Data mesh is built on the principle of democratizing data access and ownership. Domain experts who lack technical skills (such as coding or SQL proficiency) would struggle to interact with – and contribute to – the data ecosystem. This may limit accessibility and the potential for domain teams to create and manage their own data products.
Therefore, a successful implementation should provide domain teams with easy-to-use tools and interfaces that enable them to interact with data without having to write complex queries. Data virtualization-enabled solutions like the Denodo Platform abstract the technical complexities of SQL, enabling users to focus on understanding, transforming, validating, and using data rather than learning a new language. This way, domain users can effortlessly create virtual datasets, combining and transforming data from various sources without getting bogged down in coding intricacies.
Collaboration between technical and non-technical teams is essential, so collaborative capabilities and a user-friendly interface would be necessary, to encourage collaboration and a shared understanding of data.
Empowering Domain Experts
By democratizing data access and manipulation, organizations can foster a culture of data-driven decision-making, enabling business users to explore insights independently and make informed choices.
Compared to other tools that demand an understanding of SQL or complex scripting languages, teaching domain experts how to utilize the Denodo Platform is a much smoother process. Training sessions can focus on practical scenarios and real-time data manipulation, rather than delving into syntax and coding nuances. This streamlined training process not only boosts user confidence but also accelerates the adoption of data mesh within the organization.
A Data Catalog is a critical component when implementing a data mesh. It serves as a central repository of metadata, information, and documentation about the datasets and data products distributed across different domains within the organization.
Unlike traditional data platforms that often require writing complex SQL code, the Denodo Platform empowers users to develop their own data products without having previous knowledge of the underlying data sources and source-specific languages. Also, users would be able to navigate and query data using a visual approach through the Denodo Data Catalog, which helps technical and non-technical users to discover relevant datasets and to understand their quality.
Enhancing Cross-Domain Collaboration
Aligning technical teams with business objectives would be another challenge in a decentralized implementation.
The Denodo Platform bridges both worlds by providing a common platform that everyone can understand and contribute to. Business users can define their data needs using familiar terminology, while technical teams can design the underlying data infrastructure to meet those requirements and apply the necessary optimizations. This seamless collaboration ensures that data products are aligned with business goals, leading to more impactful insights and outcomes.
Changing the Game
In the era of data mesh, implementing a scalable and efficient data infrastructure is critical for organizational success. The Denodo Platform is a game-changer, simplifying data mesh implementation through its user-friendly interface and bridging the gap between business users and technical teams.
By empowering business users with the ability to manipulate data without extensive coding knowledge, the Denodo Platform accelerates data mesh adoption and fosters a collaborative environment in which insights are readily accessible and decisions are driven by data.
As organizations embark on their data mesh journeys, the Denodo Platform will provide a reliable way to unlock the true potential of their data assets.
- Data Mesh: Challenges and Solutions - October 19, 2023