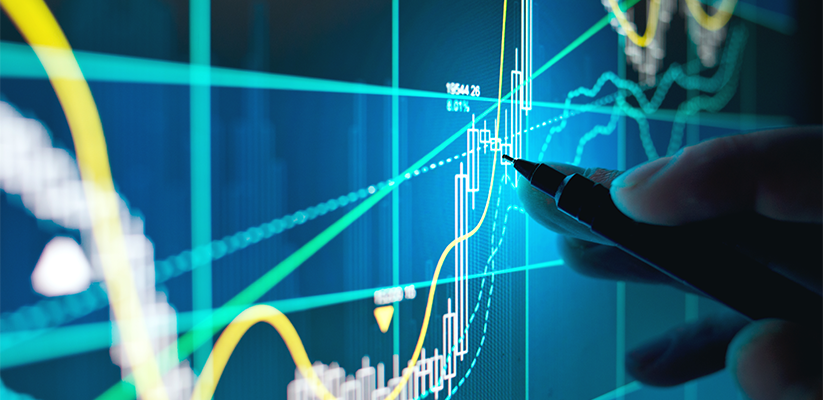
The COVID-19 pandemic and the ongoing war on Ukraine have directly impacted the global supply chain, resulting in bare shelves, long lines, unavailable necessities, and higher prices. Fortunately, the supply chain is now showing signs of recovery. On February 8, the United States White House released a two-year supply-chain report card that announces significant improvements, including the reduction of backlogged, anchored U.S. vessels from a peak of 155 to about a dozen in May of this year.
We learned a lot in a very short time. Retailers, healthcare providers, and other in-person-centric institutions quickly pivoted to remote, online business and service models. IT departments that relied solely on on-premises systems and their own managed data centers quickly learned to rely more on cloud-based systems. We learned that agility and resilience really are key, because no matter what technologies we employ, it had better be able to support multiple, often unexpected use cases; it had better recover quickly from any type of outage; and it had better be able to interoperate with myriad other technologies.
Are We Ready?
The fact is many organizations are just not ready for that next disruption – a data and analytics-based disruption, as supply chain leaders are increasingly becoming more dependent on data-based supply chain decision making. Many keep some data on-premises, due to recent mergers and acquisitions (M&A) activity, to comply with regulations around the export of personally identifiable information (PII), simply to preserve the organization of key datasets, or for dozens of other reasons. In these scenarios, it is necessary not only to maintain these different systems, but to facilitate seamless integration between them. Unfortunately, this is not easy using traditional data management approaches.
Traditionally, organizations integrate systems via batch-oriented extract, transform, and load (ETL) processes built around a central data warehouse. This strategy has been showing its disadvantages, especially in recent years. ETL processes are powerful for moving thousands of rows overnight from one source to another, transforming the data along the way to fit the target’s schemata. But they cannot easily support small, ad-hoc, real-time use cases, such as looking up one single customer’s activity in the last hour. Also, traditional data warehouses are built for highly structured data, and they cannot accommodate unstructured or streaming data sources. To support any new data sources, individual scripts need to be re-written, re-tested, and re-deployed. In short, the traditional data warehouse architecture, supported by ETL processes, just does not support the agility and resiliency needed for that next disruption.
The Data Warehouse Revisited: The Logical Data Warehouse
Analyst organizations have been studying this challenge for many years, and have proposed the logical data warehouse to surmount the challenges of the traditional data warehouse. In contrast with the traditional data warehouse, which relies on physically collecting data via networks of ETL processes, the logical data warehouse logically connects to disparate data sources, including not only structured data but also unstructured and streaming data, without replication. Because of this, logical data warehouses can connect to virtually any data, including data from just one or two records as part of an ad-hoc query, in real time. Logical data warehouses can connect to a staggering array of different data sources, such as on-premises databases, cloud-based repositories, Internet of Things (IoT) sources, data lakes, data marts, and even traditional data warehouses.
How to Begin?
Gartner recently released a report entitled “Adapting the Logical Data Warehouse for the Supply Chain” (Gartner ID #G00760575). Informed by a wealth of client input, this report provides a structure for implementing a logical data warehouse within an organization that regularly works with the supply chain. It covers how to promote interoperation with four key infrastructural components (operational data stores/operational intelligence, data warehouses, data lakes, and data science systems), how to structure the logical data warehouse to meet the needs of four key stakeholders (casual users, analysts, data scientists, and data engineers), and how to begin the process of enhancing a logical data warehouse with an organization-wide data fabric.
This report is your “Getting Started” manual for successfully implementing a logical data warehouse for the supply chain.
- For the Fifth Year in a Row, Denodo Was Recognized as a Leader in the Gartner® Magic Quadrant™ for Data Integration Report - March 27, 2025
- Denodo’s Predictions for 2025 - February 6, 2025
- My Reflections on the Gartner® Hype Cycle™ for Data Management, 2024 - December 20, 2024