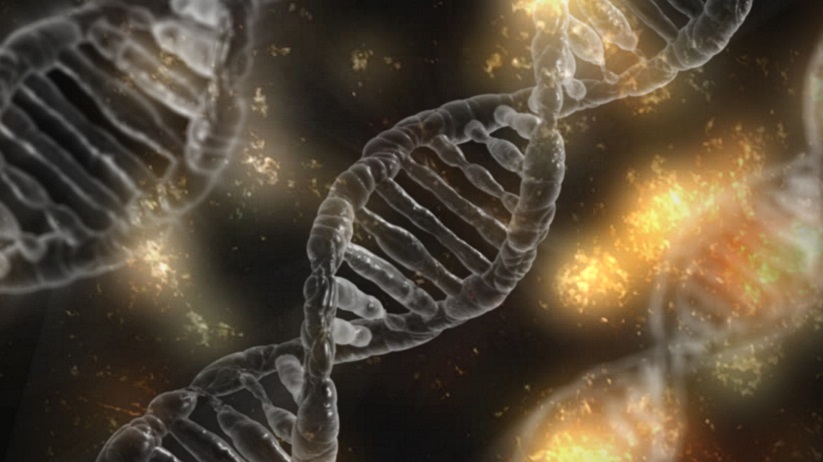
It might seem hopeless to pour buckets of money into finding a cure for a seemingly incurable disease – until it isn’t. After years of diligent effort, biopharma companies could discover the cure for diabetes, Alzheimer’s, or even cancer, and save millions of lives. Investors are extremely interested in biomedical research because they are banking on that single groundbreaking discovery. As we enter the age of genomics and genome specific drug discovery, venture and medical research firms are focusing on genome sequencing and analysis. To support this research, which involves an abundance of experimentation and observation, organizations are turning to computational power in the form of machine learning, a special kind of artificial intelligence.
Artificial intelligence is eating the world
Marc Andreessen famously proclaimed that software was “eating the world,” but there is so much more to the picture, today, since exascale computing relies on software and hardware working in tandem. In the MIT Technology Review, Jensen Huang, CEO of NVidia, boldly stated, “Software is eating the world, but artificial intelligence is going to eat software.” Machine learning is one of the many applications of artificial intelligence (AI), which interprets an aggregation of data, no matter how unique, and extracts valuable insights.
Collaborative effort in machine learning
As numerous different labs, universities, and organizations delve into this biotech industry, data piles up. From the primary data in universities to the biomedical sample data in biobanks to even the drug data within pharmaceutical companies, data is stored in various entities that might not be tied together. To keep track of their progress, researchers need a way to unify and interpret this scattered data. They must collaborate in specific forms, including innovative partnership models, customer engagement, and trust in data, so everyone is on the same page. They must also leverage cloud based bioinformatics and big data based bioinformatics, which can create an atmosphere in which all stakeholders can seamlessly access the data and process it to meet their needs.
Machine learning, also known as deep learning, requires a detailed understanding of the industry. In addition to basic anatomy, complex genome sequences, cell structure, and organ structure, machine learning must also incorporate external environmental factors such as patient demographics and drug interactions with affected cells. A big data platform or a cloud platform on its own may not be able to work with the complexities of the biotechnology industry. Instead, the most effective machine learning platform must integrate these disparate data sources to provide easier access to these various types of data.
The necessity of a flexible, agile platform
Data virtualization provides the perfect foundation for integrating these data sources. For instance, BioStorage Technologies leveraged data virtualization for its exceptional ISIDOR platform, which delivers information and data that is critical for the genome-specific treatment of life threatening diseases.
This platform consists of three main components: BioInventory, BioConnect, and BioInsight. Using data virtualization, this platform ensured that these components were compatible with one another despite their unique functions. BioInventory is a secure, cloud-based, Web-accessible storage system for global sample inventory data. BioInsight is an intelligent visualization and reporting tool. BioConnect provides the link between these two by using data virtualization to deliver sample inventory and research data to any authorized location around the world. The ISIDOR platform gathers disease/drug specific research data from universities and other research facilities, genome specific data from sample management facilities around the world, individual patient data and their demographics from other standalone systems, and applies machine learning to make clinical trials significantly more effective. Using machine learning, BioStorage can detect differences in individuals that can improve therapy decisions and success.
Machine learning and beyond
As companies invest more time and effort into big changes in the fast-paced biotechnology industry, it is imperative to address any concerns regarding privacy, security, and governance, to ensure a strong bioinformatics foundation. Here again, data virtualization plays a key role. Because it establishes a single access layer to myriad sources, it streamlines security and governance activities. By securely integrating a variety of modern data platforms, such as big data, cloud, or exascale computing, data virtualization is poised to provide the most effective foundation for machine learning, significantly changing the way the biotechnology industry works with data and facilitating a brighter future.
- What’s Needed for an Effective Renewable Energy Management Solution (REMS) - April 23, 2024
- Modern Data Solutions for the Supply Chain - April 11, 2024
- My Understanding of the Gartner® Hype Cycle™ for Finance Data and Analytics Governance, 2023 - March 28, 2024