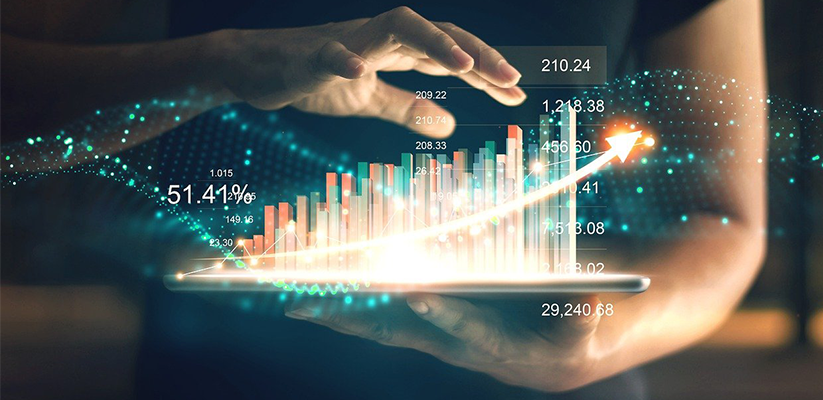
In this age of data, understanding how to harness the full potential of your data assets is crucial for making informed, data-driven decisions. This journey involves the exploration of various analytics categories, each with its unique purpose and complexity. In this post, we delve into the four tiers of analytics – diagnostic, predictive, prescriptive, and cognitive – to guide you on a path toward more advanced, proactive decision-making.
Building on a foundation
Before stepping into analytics, we need a strong foundation. To build that, we would start where many organizations start; expanding our operational reporting practice to build an analytics practice. This reporting, sometimes tagged as “descriptive analytics,” isn’t truly analytics yet, as it does not draw actionable insights, but instead provides historical observations of the data. It focuses on what happened, leaving interpretation up to the user. While essential for visualizing business facts like revenue reports and sales measurements, this is not sufficient for making data-driven, forward-looking decisions. From the perspective of the analytics practice, this is where we make sure we have accurate data in adequate volumes on which we can first build our analytics. Transparent data lineage and clear sources of truth are critical to ensure this accuracy.
Diagnostic Analytics: Uncovering the ‘Why and How’
Building on the descriptive data foundation, diagnostic analytics strives to explain the “why and how” behind observed patterns. They look for causes behind mysterious or unexpected data trends or anomalies, which requires the ability to align a variety of data assets to identify correlations and potential causation. It’s all about uncovering the hidden reasons behind your data.
Predictive Analytics: Looking to the Future
Predictive analytics takes a step further by anticipating future events based on statistical modeling, usually involving machine learning. Historical data is used to forecast future occurrences, providing insights into how business decisions can influence outcomes. These assets require careful tuning and monitoring to ensure alignment with evolving usage patterns.
Prescriptive Analytics: Defining What Should Happen
Prescriptive analytics is a more complex tier, using the output recommendations of machine learning models and historical statistics to define what should happen based on a decision. Where predictive analytics anticipate what is likely to happen, to guide human decision making, prescriptive analytics aim to make the decision for us, enabling machine learning models to take business action. This demands large volumes of data and specific models tailored to analysts’ unique needs. Such analytics generate constantly evolving patterns, and successful prescriptions may lead to reusable patterns that benefit the organization.
Data assets for prescriptive analytics often differ from those in other categories due to the complexity and volume of data required. They must be clearly labeled and isolated, often accessible only to experts. As prescriptive analytics mature and prove their value, they may generate new data assets suitable for broader use across the organization.
Cognitive Analytics: Enable the Machines
Building on the machine learning modeling of prescriptive and predictive Analytics comes the notion of cognitive analytics, where artificial intelligence algorithms are given access to massive volumes of often unstructured data to seek connections that may not be visible to human analysts, providing “cognitive augmentation” to the analytics team by suggesting patterns and decisions that may have otherwise gone undiscovered by the human teams. This type of ensemble, sometimes termed “human/cog”, builds upon the other tiers of analytic tiers to provide business-centric insights for powerful, proactive actions.
In this world in which data-driven decisions are king, it is critical to understand and harness the power of these analytics categories. By incorporating these tiers into your self-service analytics strategy, you will not only help your organization to establish or strengthen a culture of data-driven decision-making, but also enable your organization to transition from a reactive decision model to a proactive one. Embrace the data, and empower your organization to thrive in an increasingly data-centric world.
- Elevating Data Integration: A Four-Tier Approach to Effective Data Preparation - September 12, 2024
- Unlocking the Power of Analytics: From Diagnostic to Cognitive - August 16, 2024