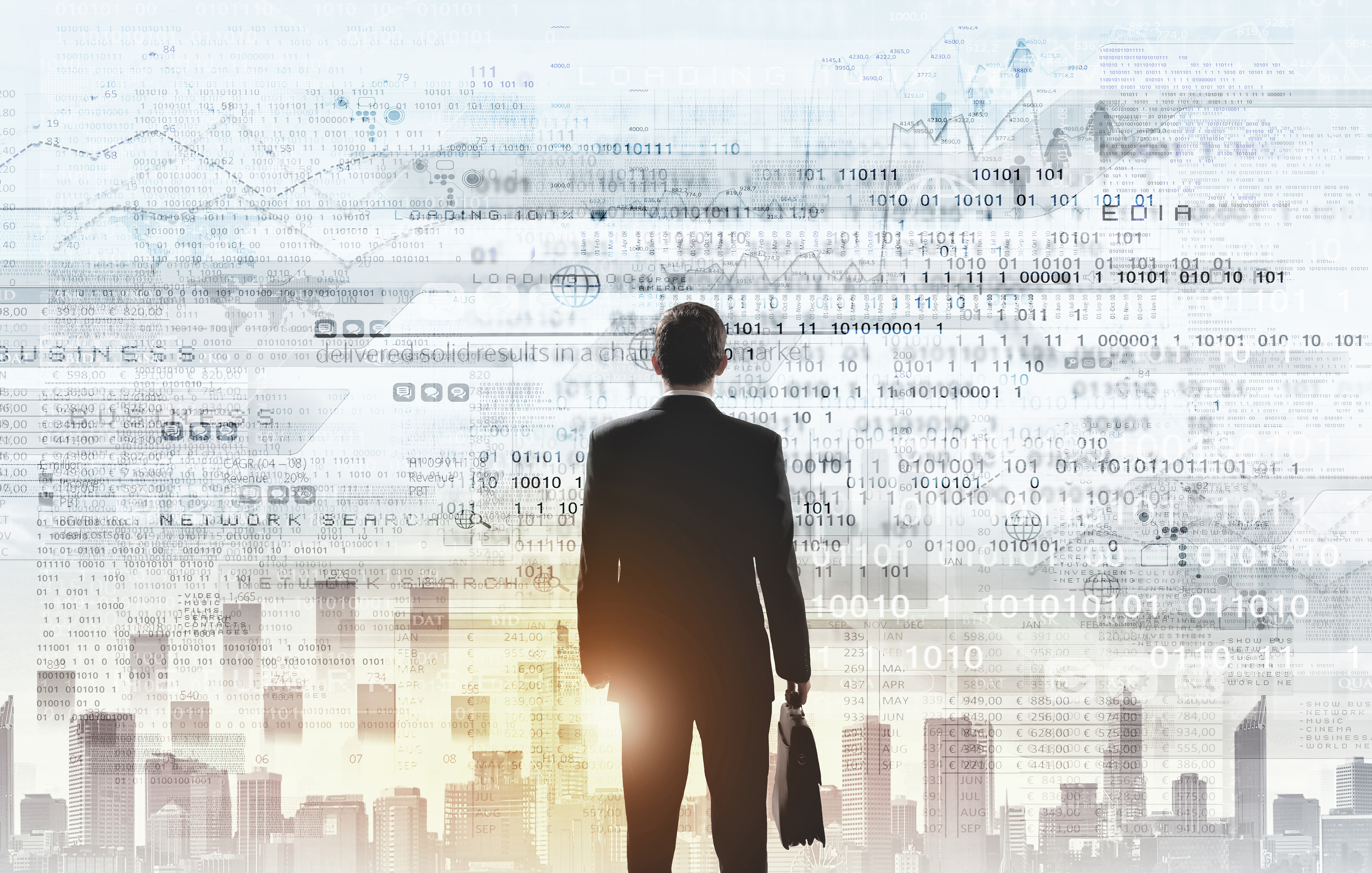
Business Intelligence (BI) is critical for the success of today’s enterprise. However, many companies still struggle to figure out how to use analytics to take advantage of their data. It is not the informational BI tools they have; it’s simply the inability to rapidly and efficiently integrate the volume of data they receive from all directions.
Most companies today are driven by data, or to be more precise, by information that is extracted from data. However, according to Gartner, “by 2017, 33 percent of Fortune 100 organizations will experience an information crisis, due to their inability to effectively value, govern and trust their enterprise information.” The problem is that, while business people get enchanted with the glint of business intelligence products such as dashboards and data visualization, they don´t realize that successful analytics depends on how well the information is prepared beforehand.
Data needs integration, design, modeling, architecting and more, before it can be transformed into consumable information for business intelligence tools. However, data integration for BI is a challenge because in order to achieve common views of business assets for information analysis, data has to be extracted from operational systems and then transformed, transported and finally loaded in a data warehouse environment.
“According to a 2015 Ventana Research survey, getting the data and getting it in order were the two biggest challenges on predictive analytics initiatives mentioned by respondents. 44% said preparing data for analysis was the top hurdle in their organizations, while 22% pointed to problems in accessing data”
The thing is, these integration methods, based on physical data movement that became mainstream in business intelligence and data warehouse contexts before the actual digital avalanche, are no longer 100% sufficient. Extracting and copying data manually from operational systems into the data warehouse is a very slow process. IT departments heavily rely on global data schemas and traditional extract, transform and load (ETL) tools that can’t handle the volume and variety of data sources that exist in large organizations today. According to Michael Stonebraker, a database pioneer and MIT professor, “a global data model is a fantasy” for organizations. “Related ETL approaches have proved to be labor-intensive, unmanageable and non-scalable,” added Stonebraker in a recent MIT Information Quality Symposium.
When Information Demand Became Greater Than Supply
Now, with such manual data integration processes, how can companies assimilate the growing torrent of data inside and outside of the organization and get any competitive advantage from analytics?
Not very easily. Traditional data integration and data warehouse approaches based on constant data movement and replication end up as BI projects that take longer to implement than they should, perform poorly or do not work at all. By the time business users make use of any integrated view for business intelligence purposes, the analytic needs may have changed, making that “information” outdated.
Indeed, instead of facilitating an integrated information environment, these traditional integration approaches have caused the growth of data silos which amplify the problem instead of solve it. The story may sound familiar to you: since data integration has been so slow in recent years, managers decided to take shortcuts to get the information they needed by generating alternate and isolated reporting solutions, such as spreadmarts or data shadow solutions.
These data shadows, created by business units on their own, have created an accidental data architecture in most organizations consisting of hundreds of data silos that are extremely difficult to manage and integrate in a fast and cost-effective way for analytical purposes. What further complicates the integration of this “spaghetti architecture” are the new data sources which need to be included in the set of analytics. Beyond pulling data from their own internal sources (which of course include Cloud and Big Data), organizations must reach into the universe of websites, sensors, customer email messages and social networks bombarding them from all directions.
Companies Running Around Like Headless Chickens
This accidental data architecture and the use of manual data integration approaches is a strategic business problem not yet recognized by C-level executives.
Nevertheless, business myopia exists. As Rick Sherman explains in his book, Business Intelligence Guidebook – From Data Integration to Analytics, all the data shadow systems mentioned before are created by individuals using different sources and criteria for defining metrics in the organization. Every department minds its own business and uses its own spreadsheets for reporting and analysis, often times using data from isolated departmental applications. Little by little, each group begins to use slightly different definitions for common data entities, such as “customer” or “product”, and apply different rules for calculating values, such as “net sales” and “gross profits.” If you add company acquisitions or global operations in different languages and currencies, you have a perfect case of data-quality shock. Put all of this finally into the environment of automated working, and we have a recipe for disaster. What does all of this mean from a business management perspective? Business users have created fractured and subjective views of the enterprise so that everyone could have their own (narrow) versions of the truth.
The information is not consistent, relevant or timely when viewed across the entire enterprise either. Should you require a common or integrated view of important company assets, such as customers, services, products, channels, markets or performance management, the IT department will most likely be unable to present it in a fast manner using a physical data movement approach. Where is the business intelligence then?
“If you can’t measure something, you can’t understand it. If you can’t understand it, you can’t control it. If you can’t control it, you can’t improve it.” (H. James Harrington).
Thus, since no one can achieve a full, connected and rapid view of the company’s performance along the entire value chain, meaning business is lacking information to truly understand internal capabilities, stakeholder’s needs and business performance, any strategic business plan for the coming year(s) will be based on false assumptions and inconsistencies. How can companies get these insights with fractured and isolated views of their business?
Towards a Logical Data Warehouse: Improving Agility of Analytic Processes
Since C-level executives and business managers are only exposed to the surface of BI projects (visualization, dashboards, etc.), they aren’t aware of their true foundation – data integration.
BI should enable business to make decisions and take actions that will create business value, but as we have seen, this process cannot be properly achieved due to the lack of consistency in the underlying data in most organizations today. While business strategies and functional tactics are discussed in board meetings every day, most decision makers are not aware that poor data integration for BI infects all downstream systems and information assets, increases costs, puts in danger customer relationships and causes imprecise forecasts and poor decisions. Some don’t even realize that they may actually be attending board meetings with wrong, partial or outdated information. Since each department has different requirements and goals, and information is housed in different data silos, it’s practically impossible for a data warehousing environment to meet an entire company’s analytical needs, neither vertically nor horizontally.
Organizations will continue to suffer from increasing business myopia if they don’t treat data quality as a strategic goal. Our claim is not that enterprise data warehouses and ETL processes are not valid. Data warehousing is a fundamental part of any company information management strategy; however, nowadays something else is required, in parallel, to boost these technologies and make data-flow rich and fast throughout the organization. Something that could make the physical location of data irrelevant as long as it is immediately accessible, integrated and presented to the BI users near real-time. An enterprise access layer that could reduce the need for data movement and make the data warehouse a logical concept, not a physical one.
Who knows, it may be asking too much. Or maybe not.
References:
- Rick Sherman: Business Intelligence Guidebook – From Data Integration to Analytics
- Rick van der Lans: Data Virtualization for Business Intelligence Systems
- Michael Stonebraker: 2015 MIT Information Quality Symposium
- Ventana Research: 2015 Value Index for Analytics and Business Intelligence Report
- Data Movement Killed the BI Star - May 12, 2016
- Data Silos: Beat the Gremlins! - October 28, 2015
You articulated the problem very well, and for anyone that has worked at a Fortune 500 company will know, you are addressing a well known problem that is pervasive in corporate America. In my opinion, the whole concept around data warehousing, and ETL is the root of the problem. Data is moved from silo to silo without any visibility of the data across the enterprise. Nobody has a good handle on what data is being moved; where is the data coming from, where is it going, and why?
While a global data model might be a fantasy, the logical flow of data through the data pipeline requires that at some point the data be understood. Understanding the data is paramount before any meaningful analysis can be performed. One of the key components on the journey to understanding data is the model. Entities must be defined, attributes assigned, relationships understood, and business rules and logic enforced. So, if the global data model is a fantasy, an industry specific enterprise model cannot be a fantasy. Barring a well-thought-out data architecture requires an enterprise view of the data. My personal belief is that most of the large corporations are already experiencing the the information crisis you mention.
So you articulated the problem well, but what is the solution?
Hello Randall, thank you very much for your feedback. I am glad that you share our vision about the data fragmentation problem that large companies face today, and how it impacts on company revenues, margins, and organizational efficiency.
Answering your question, in order to solve data fragmentation pains we advocate an enterprise view of data achieved through data virtualization. In your comment, you mention the need for an enterprise data model where the business entities and rules can be defined and enforced. Data virtualization adds just that: an enterprise-wide metadata layer in which you define the data model according to your business needs; this layer is executable and it can therefore enforce your business rules. From this metadata layer, you can point to wherever the data is, eliminating the need to replicate the data and providing agility and better business insights through the integration of many data sources.
As you mentioned, moving the data from silo to silo does not provide any visibility and it is never a complete solution. Continuously copying data is not sustainable anymore. You are always late to the party, I would say! Data virtualization can complement and boost data warehousing and ETL technologies to facilitate broader and faster data integration scenarios across the enterprise by building a sort of “Logical Data Warehouse” abstraction layer where there is no need to transform and move data all the time. That´s why I ended the blog “wondering” about a data integration concept that could make the data warehouse a logical concept, not a physical one. And that is data virtualization…
I invite you to watch in this link (http://goo.gl/BN3cqs) a recent video from Mike Ferguson (Independent Analyst) where he explains these concepts of data virtualization and Logical Data Warehouse and how it improves business performance and agility while reducing time to value in a company. Hope it helps. If you have more questions you are very welcome.
Thanks again!