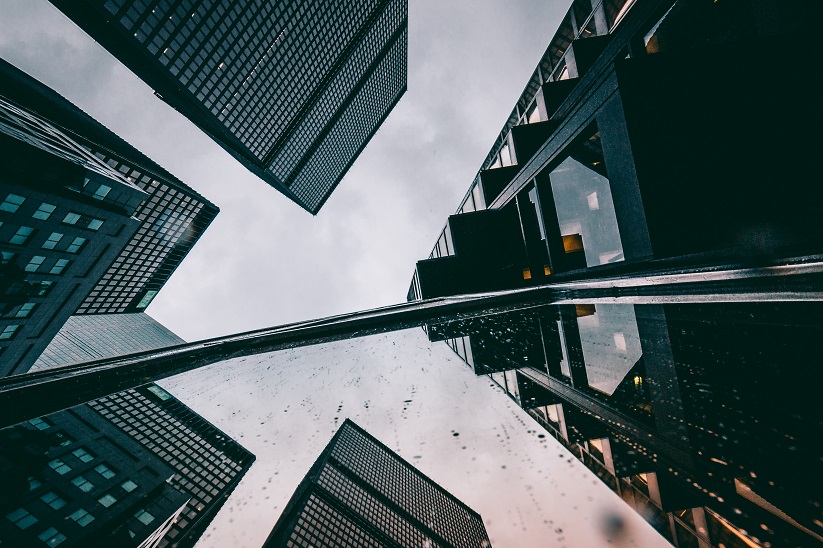
This is the time of Kin-directed IoT resource sharing. It is an era of AI nation that carry on a cooperative behaviour and inhibit dispersal of resources in an Internet of Things environment; such as, Cameras, drones, cars, and wearables. Moreover, we as humans will provision this collaboration based on mutualism since all will receive an immediate payoff for cooperation.
To illustrate, the collaboration with the closet kins will happen based on spatial proximity and associative learning thanks to emergence of technologies like AI, edge computing and 5G. Citizens in the AI nation will walk down the streets of an augmented city with wearable devices that monitor their health and send them real-time alerts. They will share the data with smart nearby devices like CCTV cameras, drones, and vehicles on the streets. These devices will reward them with bigger computational power than their tiny wearable can achieve. In addition, cameras will receive contextual data from us and they will provide video streams to vehicles and receive from them computational power. Hence, vehicles with such data may be able to find the optimal routes and avoid traffic jams. It is the harmony and collaboration of humans and machines that make people’s life better.
IoT applications spans different computational capabilities from low-end devices like wearables to high-end devices like VR and drones. New paradigms, standards, and technologies emerged recently to orchestrate an intelligent resource allocation of numerous IoT devices so that each device could collaborate and contribute in provisioning services. Such resource allocation paradigms were present in mobile cloud that carry on computing use cases provisioning computation, storage and networking cloud services between mobile devices and remote cloud data centers. However, such paradigms cannot fulfill the latency-sensitive and bandwidth requirements of new emerging IoT applications since they require a communication with a remote cloud server. Also, IoT use cases requires collaboration that utilizes sensing, location-based services, and actuation with the upcoming need for latency-sensitive and mobility challenges. Furthermore, new paradigms allow the exploitation of 5G radio resources and edge computing to facilitate the collaboration of myriad IoT devices across not only in a city neighborhood scenarios but also in a city-wide scenarios. This would result in smarter usage of resources and open up the possibilities for new use cases in forthcoming 5G access segments at the edge of a cellular network.
In addition, IoT Gateways acts as a bridge that provides the passage between the edge and cloud allowing data pre-processing and aggregation before it is transmitted to the cloud. These gateways should act as low-latency aggregators of data coming from distributed end devices/sensors. They could also perform persistence storage. In order to have a more efficient data management practice, data virtualization could augment with IoT gateways by providing real-time data integration, thus, facilitating new innovative analytics use cases at the edge. Indeed, it is a cornerstone to make the “Kin-directed IoT resource sharing” paradigm possible.
To conclude, for us, humans, reward-based associative learning may not be enough to share our resources with robots and machines. However, as robots or ubiquitous speech-activated devices like Alexa or Google Home become more anthropomorphistic embodying humanistic traits from voice tonality to design, convenience, sociability, and experience, we will witness an increase in people’s affection towards such devices and robots. Thus, a cultural shift might take effect to enable such Kin-directed IoT resource sharing.
In my upcoming posts, I will cover the other dimensions of the “Kin-directed IoT resource sharing” era and I would be happy if you share with me the challenges of such paradigm in the comments section.
- If Trust is the Main Ingredient of Leadership, Is Trust the Main Ingredient of Successful AI? - November 18, 2020
- The Main Pillars of Future Polymer Factories, the Next Future Material for AI - November 15, 2019
- From the Bronze Age to Today’s AI-based Circular Economy, and How Data Virtualization and Federated Learning Make It Possible - August 13, 2019