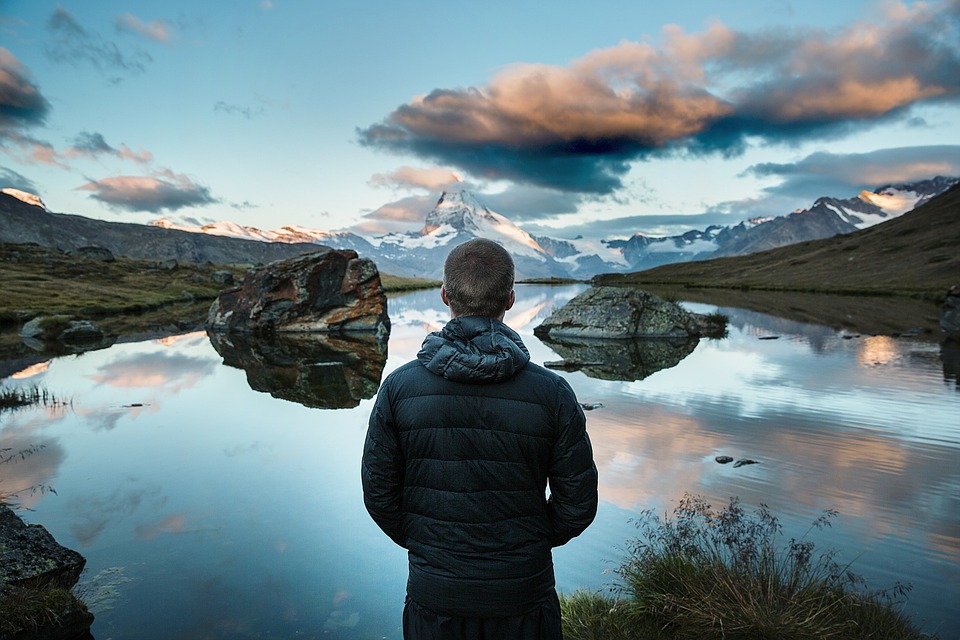
Imagine you’ve been walking in the Alps on a hot summer’s day, you’ve reached the highest point of your trek and you come across the crystal clear water of a mountain lake, who wouldn’t want to jump in? In the UK where I live, it’s rarely hot, there aren’t many mountains and the nearest ‘lake’ we have is an old local quarry full of murky brown water. Unable to see the bottom, jumping in and ending up with my bare feet squelching through the muddy lake floor, getting caught in slimy weeds or a local supermarket shopping trolley (discarded after a night of student revelry) by contrast doesn’t quite have the same appeal, no matter how hot the day!
The Murky Depths of the Ungoverned Data Lake
This contrasting uncertainty becomes yet another water based metaphor (sorry) for the challenges facing companies wishing to ‘take the plunge’ into a data lake architecture that allows all their data to be accessed in one place. On the one hand the data lake promises to solve the problem of traditional ‘data silos’, acting as a hub to allow consumers to dip in and get the data they need. However, as my colleagues have described in two posts last year, there are some problems with this approach.
Paul Moxon, in his blog “Logical data lakes”, discussed the challenges of getting all your data into a physical lake in the first place. Most companies have many different data repositories and it’s simply not realistic to load all of this data into a central repository (the ‘data lake’) in one go and give everyone access to it. Paul also discussed how data virtualization can support a logical data lake, allowing data to remain in the data sources initially and transition to replicated data lake architecture over time if required.
Pablo Alvarez, in his blog “Avoiding the Swamp: Data Virtualization and Data Lakes” discussed the risks to the success of a data lake and the potential ‘data swamps’ that result. Pablo points to Gartner’s article “Beware of the Data Lake Fallacy” which suggest that the biggest risks to a data lake are by definition due to the inherent lack of governance, consistent security and access control afforded across all the data in the lake. Typically data can be placed into the data lake with little thought to its contents and the company’s privacy and regulatory responsibilities. Gartner propose that a lack of governance and the the inability to understand the quality and lineage of data in the lake severely reduces a company’s ability to locate data of value and reuse it.
So without knowing what data is in the lake who wants to go swim in them, just like my murky local quarry?
The Crystal Clear Waters of the Governed Data Lake
Recently I’ve been seeing a trend of companies wishing to benefit from a data lake architecture but who are looking to steer away from the un-governed ‘free for all’ approach and instead develop a governed data lake.
These companies are designing in governance from the start, and are taking a more conservative approach to their data lake architecture. Rather than dumping all data into the lake, the governed data lake only allows ‘verified’ data to flow into it. These flows apply standardization rules, transformations and aggregations to the data so that it can be consumed with confidence from the data lake by all users.
Typically we see that a governed data lake architecture aims still to not restrict the types of data structures that are stored in it. The key thing is to ensure that no data is stored in the lake without documented business context and certification, so that the data in the lake becomes a re-useable and valuable asset, no matter what the format. Consequently, we see that companies implementing governed data lakes are still expecting to store their data in its native structure which means that rather than a being a single physical repository, governed data lakes still comprise multiple data repositories e.g. a combination of RDBMS, Hadoop, NoSQL, files (XML, JSON, CSV).
To provide a layer of governance, metadata management and business glossary tools are being employed on top of the data lake repositories to define the business meaning of the data and location of the data in the governed data lake. Only data that is linked to corporate definitions in this governance layer can be published to the governed data lake consumers. This obviously requires a governance process to support it, but while these tools define the meaning and location of the data, a challenge still remains – how to make the data easily discoverable and accessible while providing a consistent security model across all data items irrespective of the data repository in the data lake?
Consequently, companies are turning to data virtualization to complete their governed data lake architecture, by providing a common access and security layer, and search and discovery capabilities. This is a great validation of Denodo’s most recent platform release, Denodo 6.0 which became generally available at the end of March 2016. Denodo 6.0 includes a new web based Information Self-Service Tool expressly designed for navigating the data virtualization layer and discovering the data that’s securely published by it.
Data Virtualization Helps Navigate the Governed Data Lake
In the case of a governed data lake, data virtualization’s role is not just to provide performant and complex combinations of data across multiple data lake repositories, but rather primarily to act as a consistent mechanism to allow all types of user to discover and access all the data in the lake, whether they are BI users, Business Analysts or Data Scientists. Data virtualization provides several benefits in this context.
It facilitates the publication of the data in the governed data lake via multiple models without having to replicate the data. This means the same data can be presented in both physical and business context, allowing consumers to access data more intuitively by business definition (as defined in the governance tools), or via physical attribute (e.g. table, column name) depending on their role in the organisation. So, whether you are a BI Analyst wanting to locate appropriate business data for a MI report or a Data Scientist wishing to locate data for analytics, users reuse the same data in the lake but in a context that is most meaningful for them.
Denodo 6.0’s Self-Service Information Tool allows users to search for both data and metadata in the virtual layer. This means in the context of a governed data lake, users can locate data views via keyword search based on business terms or physical attribute names. They can then query the located views through the same browser interface to verify the data is relevant for their needs.
Another benefit of publishing the governed data lake’s data via data virtualization is the ability to view the relationships between entities published in the data lake – both physical and business. Denodo 6.0’s Information Self-Service Tool provides this information graphically; once users have located relevant data entities in the data lake they can then locate more intuitively other related entities and data of value.
Perhaps the final and most important benefit to many companies that data virtualization brings, is its ability to publish the governed data lake’s data via a consistent security model. Whether the data is being accessed via logical or physical models or via any access tool, e.g. via a browser, external SQL query client, BI tools, statistical analytics packages or even data preparation tools. In all cases, consistent user and role based security privileges are applied, ensuring that the data from any repository in the governed data lake be it an RDBMS, Hadoop or other, is only seen by those with the correct authority.
Governed Data Lakes – The Future?
Data virtualization is being accepted as a key enabler of governed data lakes because of these benefits. We are even seeing organizations experimenting with auto-generation of a data virtualization layer directly from the metadata defined in the data lake governance tooling. The future goal being the fully automated end to end publication of governed data and associated business metadata in the governed data lake.
Time will tell, but data virtualization appears to be cleaning up the waters of the data lake and making the prospect of taking a plunge more inviting.
- Data Virtualization: Thinking Outside the Bowl - July 31, 2019
- 3 Pillars of GDPR Compliance - May 9, 2018
- 3 Steps to Data Protection Compliance (GDPR) - May 9, 2017
Awesome post! A very good step-by-step guide especially for a beginner like me. It’s overwhelming with information, thank you for making it easy and very detailed.. I’ll pop some questions here, if I need help, hope that’s okay.
Hadoop training in Chennai
Thanks Jeeshi, glad you found it useful!
Awesome blog. I enjoyed reading your articles. This is truly a great read for me. I have bookmarked it and I am looking forward to reading new articles. Keep up the good work! corporate training