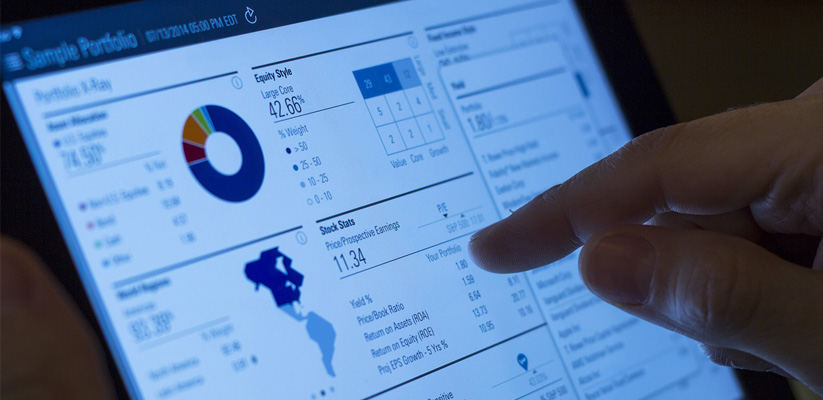
I’ve had several conversations with BI practitioners recently around the need to provision data agilely in order to support Self-Service Business Intelligence initiatives. Many have been confused as to the roles that some of the great data blending tools out there play in contrast to data virtualization technologies.
Data Virtualization promises a quick method for providing access to data, across multiple heterogeneous data sources, so that it can be easily consumed by business users, but don’t data blending tools offer the same thing? As discussed in our previous blog post “Self-Service BI and Data Virtualization”, there are more considerations than simply enabling self-service access to the source data systems.
The Basics
Perhaps we should first consider some basics.
What is Data Blending?
Data Blending tools put the ability to connect to a wide range of disparate data sources and join the data together, in the hands of the analyst. They typically provide a graphical method of defining a data preparation process flow that maps out how the data sources are to be connected, how the data from the sources are to be connected, transformed and joined through a number of physical steps. When the process is run, it physically pulls the data from those systems, applies the transformations, joins and writes the results to another data store ready for analysis.
They are designed to assist the Business Analyst in preparing an analytical dataset that is created to answer a specific business question. They are seen as a tool that can remove the dependency on IT, allowing the Business Analyst to connect to the data sources themselves, extract the data and transform it in a timely manner. They are designed to reduce the time spent by the analyst in preparing data, which is often quoted as 60% plus of the overall time spent in analysis.
What is Data Virtualization?
Data Virtualization also provides the ability to quickly connect to and combine a broad range of data sources. However, it provides a logical view on top of the physical data stores that can be easily queried by data consumers, to support self-service access to the data. It abstracts the complexity of the integration and transformation across the data sources from the consumer, combining data on the fly from the data stores to present a unified result set. It is a declarative means of combining the data rather than a defined process flow, and presents a virtual view on the data, rather than physically transforming and storing the data outside of the data sources.
Data Virtualization technologies are designed to assist IT in provisioning data to the Business quickly, speeding time to data delivery, reducing IT workload and providing data as a service across the business.
5 Key Considerations in Provisioning Data for Self Service Business Intelligence
While both technologies have their place, when it comes to Self-Service Business Intelligence there are 5 key differences:
-
- Agility – Both tools provide an agile means of data access for the user reducing the dependence on IT however, both need to be viewed in different contexts. Data Blending tools are aimed at the Business Analyst, allowing them to blend data for their own purposes. Data Virtualization while predominately an IT tool, enables IT to provision data to the Business much more quickly removing the overheads associated with physical integration approaches.
- Re-use of Data – Data Blending tools are designed to support the preparation of datasets to answer or specific business or analytical questions. Normally an analyst works with the data in silo and builds a data set for a specific purpose. Data Virtualization is designed to provide a logical model oriented view of data across a number of physical stores that can be consumed by many applications for many purposes.
- Data Movement – Data Blending tools involve physical movement, transformation and replication of the data. Data Virtualization takes the processing of the data to the data store, leveraging the processing power of the data store as appropriate and reducing the costs associated with data storage.
- Governance – Data Blending involves physically moving the data outside of the applications and data stores themselves, storing the result sets outside of the native application security domains. This adds complexity and challenges in terms of ensuring security of the data, understanding who is using the data and the dependencies on the data extracted. It can also lead to a dataset explosion if the datasets are repurposed for other uses, and all the challenges of governance that goes with it. Data Virtualization provides a central point of access to the data, and implements a common security model across the data sources. It provides data lineage and audit over who is using the data, improving governance in a self-service environment.
- Publication of Data – Data Blending typically creates a dataset that relies on a Data Visualization tool to ‘publish’ it to the consumer. Data Virtualization technologies support multiple provisioning mechanisms. The same model can be accessed by BI tools, middleware technologies, as a Web Service and can even be a source for ETL and Data Blending tools – providing governance and re-use of common business rules.
Data Blending tools are great for preparing data to help answer unique business questions and analysis. However, when considering your Self-Service Business Intelligence strategy ask yourself: do you need to share data across the enterprise? Do you need to do that with agility while maximising re-use? Do you need a governed environment? If the answer is yes then think Data Virtualization.
- Data Virtualization: Thinking Outside the Bowl - July 31, 2019
- 3 Pillars of GDPR Compliance - May 9, 2018
- 3 Steps to Data Protection Compliance (GDPR) - May 9, 2017
Excellent, concise summary.
“Data Virtualization also provides the ability to quickly connect to and combine a broad range of data sources.”
The more you can streamline operations, the better chances you have of increasing the ROI of your BI platform. Being able to quickly connect and combine multiple data sources means your business has streamlined reporting capabilities that are able to keep up with data that is constantly getting captures and analyzed in real-time.