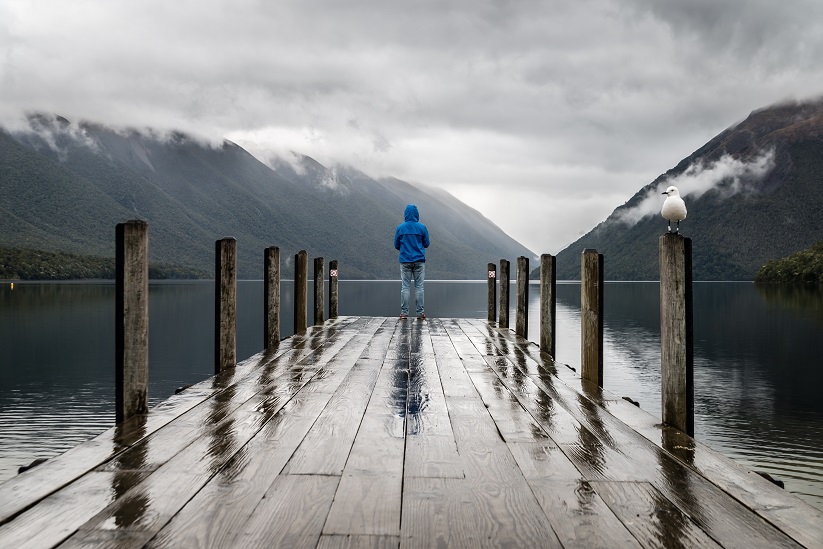
Fresh from her success in supplying real-time transaction data to the call center using the Denodo Platform, Alice Well, recently appointed CIO of Advanced Banking Corporation (ABC), hears the three familiar, demanding raps on her office door. She glances toward the heavens, whispering “all is well, all will be well.”
“Why are you waging war on my warehouse?” BI manager, Mitch Adoo (About Nothing) barges into the room. “Why are you promoting a data lake from that upstart acquisition over all careful work we’ve put into the warehouse? You know they just keep dumping data in there until it has become a stinking swamp.”
“Slow down, Mitch,” says Alice. “I’m giving you the opportunity to focus the warehouse on what it does best: the creation and management of a consistent set of core business information for the entire corporation. Meanwhile, the data lake can handle all the other, dirty, less critical data. The warehouse will be a key component in allowing business and IT folks to get to it in a well-managed and understandable way. Your work will be even more important for ABC.”
Mitch is now smiling broadly. That’s more like it!
“Read my architecture document,” Alice adds. “It’s based on our new data virtualization platform.”
The Old Way: Lake Displaces Warehouse
Since its initial introduction in 2010 by James Dixon, CTO of Pentaho, the data lake has grown in popularity as the place to store all data for analytic use. Its open source technology foundation offers advantages in cost, agility in development, speed in loading, and ease of use. This is because of its “schema-on-read” approach, which allows data to be stored in its original form rather than having to be structured into a relational model on loading (schema-on-write).
The above advantages are of most benefit for externally sourced information. However, the cost argument, in particular, has led many organizations to plan to eliminate their warehouses, although they were built mainly for well-structured, internally sourced data. Issues arise, however, in this elimination plan. Existing investment must be written off and current staff skills are devalued. Data management in data lakes remains primitive in comparison to the relational environment, a real concern in the case of financially and legally binding data.
Alice, like many thoughtful and experienced CIOs, has concluded that a better approach is to keep the data warehouse with a more focused role while digging and filling a new data lake.
The New Way: A Warehouse on an Island in a Lake
The phrase “warehouse on an island in a lake” suggests that the data warehouse is an island of stability, structure and management in an otherwise fluid and changeable data lake. The warehouse becomes both a store of core business information and a contextual reference point for all the rest of the data in the lake. The warehouse retains its relational structure, while the lake uses whatever set of technologies is appropriate.
However, business people must be able to access and use all this data, irrespective of its storage technology. They must be able to “join” data across the warehouse and lake with ease and elegance. This, of course, is where data virtualization technology is vital in implementing the approach, as shown below.
The warehouse, consisting of an enterprise data warehouse (EDW) and data marts, remains as it was. However, further growth is first reined in and its use for advanced analytics and externally sourced information may be restricted. Non-core data may be later removed. The EDW serves two purposes. First, it is the certified and reconciled source for data from the operational environment, provided to business people via data marts in the usual fashion. Second, for the data lake, it acts as reference data and metadata in support of the wide variety of more loosely structured and less well-defined data there.
Data virtualization mediates access by business people and apps to all data in the lake and data marts (and also the EDW, if required). In the Denodo platform, such mediation is built on an extended relational model and supported by a comprehensive and dynamic Data Catalog. The model of an existing EDW acts as a foundation for the extended relational model of the broader data lake.
The Denodo Data Catalog is a dynamic catalog of curated, reusable and timely information about the content and context all data available through data virtualization. Its dynamic nature arises from its direct linkage with the data delivery infrastructure, thus ensuring that its contents are always current. With the EDW data and metadata available from its island within the data lake, data stewards and business users alike can be assured that, not only is the catalog up-to-the-minute, but contains the reconciled and agreed definition of the business’ core data.
Leveraging powerful searching and browsing functionality of the Denodo platform, business people can seamlessly move between metadata and data wherever they reside, with no need to understand or construct complex SQL queries. Data stewards and administrators also benefit from the Catalog with real-time metrics of data usage, timeliness and quality in the data lake, oriented around the key business concepts and structures found in the EDW.
As Alice says, all is well and all will be well when IT can reuse and repurpose the high-quality design work that went into the data warehouse to support new platforms such as the data lake. Such reuse is only possible with a powerful data virtualization platform built around a dynamic Data Catalog such as that offered by Denodo.
- The Data Warehouse is Dead, Long Live the Data Warehouse, Part II - November 24, 2022
- The Data Warehouse is Dead, Long Live the Data Warehouse, Part I - October 18, 2022
- Weaving Architectural Patterns III – Data Mesh - December 16, 2021