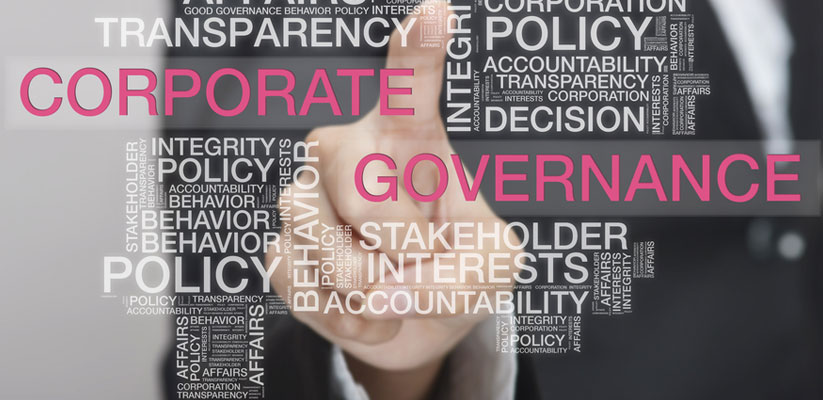
The Problem
“How do I find mouse epithelial cell lines?” “I’m looking for a mus musculus prostate cell line—does anyone have one?” “I need some CRL-2731, where is it?” While scientists may recognize that these questions could all have the same answer, most information systems would not. Biopharma research scientists waste untold hours translating between terms such as “mus musculus” and “mouse,” rather than leveraging data productivity tools, such as a defined data structure and synonym management system. While manual query translation can be done to address a single question, it fails completely when broadly mining historical research data.
This situation arises because information is treated as a local resource by scientists and project teams. Their sole focus is on finding the next target or optimizing the next compound; data is seen as something only they need to manage, not as a valuable corporate asset. Because a proper place has not been defined, data is stored wherever the scientist finds it’s convenient—in a local spreadsheet, on a shared drive, or in a notebook. The scientific information landscape ends up resembling a teenager’s room in which disorder becomes the norm because “it’s easier for me to find things that way.”
The Solution
Data governance provides a solution. If we want to organize a lab, the rule is “A place for everything and everything in its place.” Exercising data governance means coming to an upfront agreement about what things we need to track (e.g. cell lines, proteins, assays), what we will call these things (mouse vs. mus musculus), where we will store data (which system for which data), and what attributes will be recorded (e.g. chemical structure, strain of mouse, dosage). It requires agreement on the vocabulary used and the process by which new items, terms, or attributes will be added.
However, simply defining data governance attributes doesn’t suffice. Every company would have biopharma research data organized, if only frameworks, processes, and tools were required. Most data governance efforts fail because they don’t answer the compelling question of “What’s in it for me?” Ultimate success requires the hearts and minds of data generators and data consumers.
The Opportunity
Every drug discovery scientist and biopharma development team is under immense pressure to speed molecules through the pipeline. Winning the race to a patent, to an approved drug, and to the marketplace is rewarded with millions of dollars and countless lives improved. Data governance speeds scientific outcomes. It puts in place a structure to fully optimize data value by providing fast, efficient, global access—where scientists can find not only what they’ve produced, but others can find it, too.
What’s the data governance payoff? It’s a seamless web of interconnected data points that describe all aspects of a therapy—from initial discovery, to optimization, to pre-clinical safety, and ultimately, to human testing. It’s having the ability to assemble data rapidly in response to ad hoc queries, for reports summarizing program progress, and for regulatory filings. Most importantly, it’s giving your scientists the ability to make the most of the information your organization has already generated, and in so doing, quickening time-to-market of new patient therapies.
This blog was penned by David Hartsough, Senior Manager, LabAnswer
- Data Integrity in the Cloud - January 18, 2017
- Controlled Vocabularies, Taxonomies, Thesauri and Ontologies for Knowledge Management: A Primer - January 11, 2017
- Data Governance: What’s in it for me? - September 15, 2015