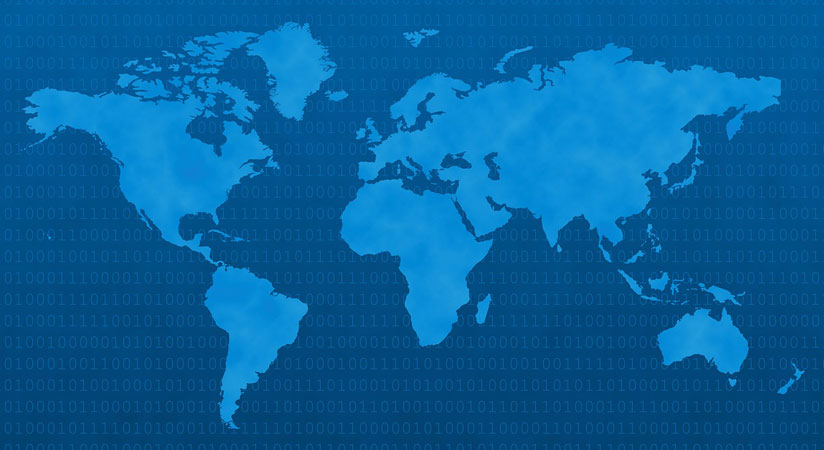
Data virtualization is being used in everything from small projects that are in need of quick data access, as part of bigger application or data warehousing project, as an enterprise-wide semantic or common data layer or any combination thereof. The use cases can also be classified as Analytical, Operational and Data Management. The white paper “High Value Uses of Data Virtualization” demonstrates how 15 different customers, across a variety of industries have used data virtualization to their advantage.
In terms of examples, let’s look at business intelligence and analytical use cases first, including what is now called “Agile BI”, the use of virtual data marts, or building logical data warehouses with data virtualization. These use cases typically start off using data virtualization for rapid prototyping or to meet an immediate business need and then evolve to a hybrid solution. Often these are used to build a real-time reporting solution that federates access to multiple internal databases, Cloud applications like Amazon WS, Salesforce or Workday, Big Data, flat files or Excel spreadsheets, etc. Real-time dashboards for operational and performance management, network and resource monitoring, etc. are also good fits for data virtualization because they too need real-time information from internal and external sources.
Big Data & Predictive Analytics are hot areas as well, but the investment in these specialized data stores and analytical processing capabilities tend to create even more silos. This is something that could be avoided if they were built on a data virtualization framework. This helps in two ways; first it delivers broader information to the analytical or Big Data stack, and second it exposes the resulting data and analytics which can be in complex formats to the rest of the enterprise in an abstracted (and easy to consume) way such as REST or SQL. This promotes their reuse within operational and day-to-day usage scenarios.
In terms of Operational uses cases for data virtualization, these often include provisioning data services for single-view applications such as in a call center or customer self-service application for a Telco operator; a unified underwriting application for an Insurance carrier; or a virtual inventory management application across a retailer and/or supply chain network. Further, virtualized data services are increasingly reused across multiple applications and business processes making them even more cost effective.
Data Management use cases are also being adopted more broadly. Companies create a unified virtual data layer, also known as a semantic or common data layer, to capture the common metadata by domain across the enterprise which is then encapsulated in canonical data services that can be consumed by both BI and operational users. Extended to master or reference data, this creates a virtual or hybrid master data management (MDM) solution. Enterprise data services that support Agile SCRUM application development practices for both SOA applications, and in newer REST/JSON outputs for mobile and Cloud applications, are becoming popular.
Finally, companies use data virtualization as an abstraction/decoupling layer to mask volatility in data sources and applications during major changes such as mergers & acquisitions, application modernization and/or Cloud/outsourcing initiatives. More specific examples of data virtualization use cases can be found here.
Read more: http://www.virtual-strategy.com/2013/06/17/interview-suresh-chandrasekaran-svp-denodo?page=0,1#ixzz2f8wxvWsg
Read more at http://www.virtual-strategy.com/2013/06/17/interview-suresh-chandrasekaran-svp-denodo?page=0,1#zkLQRshYSHCCivkX.99
- Data Governance in a Data Mesh or Data Fabric Architecture - December 21, 2023
- Moving to the Cloud, or a Hybrid-Cloud Scenario: How can the Denodo Platform Help? - November 23, 2023
- Logical Data Management and Data Mesh - July 20, 2023