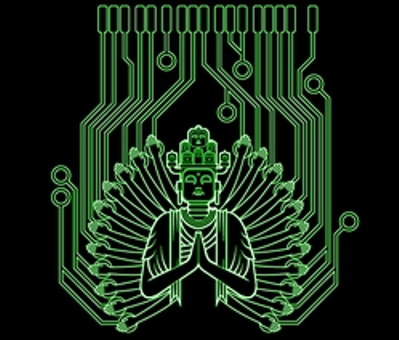
Disclaimer: The author felt compelled to share some ideas that emerged at a Buddhist Vegan restaurant in Palo Alto, CA after a conversation on big data and data virtualization with analysts from a top CIO membership organization. The author claims no expertise on revered Buddhist philosophy, or for that matter, vegan cuisine. Those who know better are advised to seek wisdom elsewhere.
Big Data is being talked about everywhere… in IT and business conferences, venture capital, legal, medical and government summits, blogs and tweets … even vegan restaurants! Given the sheer volume of such talk, one could wonder “Is this knowledge without wisdom? Action without results? Technology without business purpose? Or is it all of the above?”
My suspicions were stimulated when representatives of an exclusive CIO membership organization let me in on a secret – CIO’s are feeling pressured to do something about Big Data. So while they are putting up Hadoop clusters and crunching some data, it seems that the really big (data) question all of them have is where is the value going to come from?, what are the “real” use cases?, and finally how can they prevent this from becoming yet another money pit of technologies and consultants?
While ruminating on this in the said vegan restaurant, the image of the “wheel of life” (Bhavachakra) in Buddhist tradition, offered an allegory of rising towards a higher purpose (nirvana) by embracing life’s journey (noble path through 6 realms) to overcome ignorance and likes/dislikes (the 3 poisons ).
These ideas morphed into the three spokes on the wheel of big data life: 1. The Quest for higher purpose or business value 2. The Journey into multiple realms of data, and 3. The Conquest of the three demons of cost, time and rigidity.
- The Quest for Higher Purpose or Business Value: Much of the talk about Big Data is focused on data … not the value in it. Perhaps we should start with value – identify those business entities and processes where having infinitely more information could directly influence revenue, profitability or customer satisfaction. Take for example the customer as an entity.If we had omniscient knowledge of current and potential customers, past transactions and future intentions, demographics and preferences – how would we take advantage of that to drive loyalty and increase share of wallet and margins? Or to focus on a process such as delivering healthcare services – how would Big Data impact clinical quality, cost and reduce relapse rates? Enumerating the possible impact of Big Data on real business goals (or social goals for non-profits) should be the first step in the Big Data journey, followed by prioritizing them which would involve weeding out the whimsical and instead focus on the practical.The quest for higher purpose or business value can be achieved by the democratization of data access, giving business people and front-line employees easy and timely access to discover and play with data services (information as a service). This can lead to information nirvana (enlightenment) and shine light on new and surprisingly valuable ideas for using Big Data.
- The Journey into Multiple Realms of Data: A true seeker of value from Big Data is not afraid to venture into any kind of data or information however difficult it might be to acquire and integrate.With a burning quest for value, they open their minds and approaches to harness and experiment with many different types of Big Data (structured to highly unstructured) and sources – machine and sensor data (weather sensors, machine logs, web click streams, RFID), user-generated data (social media, customer feedback), Open government and public data (financial data, court records, yellow pages), corporate data (transactions, financials) and many more. In many cases the “broader view” may yield more value than the “deep and narrow” view. And this allows companies to experiment with data that may be less than perfect quality but more than “fit for purpose”. While quality, trustworthiness, performance and security are valid concerns, those who over-zealously filter out new realms of data using old standards, fail to achieve the full value of Big Data. Also data integration technologies and approaches are themselves siloed with different technology stacks for analytics (ETL/DW), for business process (BPM, ESB), content & collaboration (ECM, Search, Portals). Companies need to think more broadly about data acquisition and integration capabilities if they want to acquire, normalize, and integrate multi-structured data from internal and external sources and turn the collective intelligence into relevant and timely information through a unified/common/semantic layer of data. While there are multiple realms in the journey, some hard with less than perfect data, some easier with low-hanging access, if you look in the right direction with a sense of purpose, and persevere with agility, they all lead you, inexorably towards stronger Big Data capabilities and faster value.
- Conquest of Cost, Time and Rigidity: While all the data in the world – and its potential value – can excite companies, it would not be economically attractive except to the largest organizations if Big Data integration and analytics were done using traditional high-cost approaches such as ETL, data warehouses, and high-performance database appliances. From the start, Big Data projects should be designed with low cost, speed and flexibility as the core objectives of the project. Big Data is still nascent, meaning both business needs and data realms are likely to evolve faster than previous generations of analytics, requiring tremendous flexibility. Traditional analytics relied heavily on replicated data, but Big Data is too large for replication-based strategies and must be leveraged in place or in flight where possible. This also applies in the output direction where Big Data results must be easy to reuse across unanticipated new projects in the future. To prevent Big Data projects from becoming yet another money pit and suffer from the same rigidity of data warehouses, there are four areas in particular to consider: data access, data storage, data processing, and data services. The middle two areas (storage and processing) have received the most attention as open source and distributed storage and processing technologies like Hadoop have raised hopes that big value can be squeezed out of Big Data using small budgets. But what about data access and data services? Companies should be able to harness Big Data from disparate realms cost effectively, conform multi-structured data, minimize replication, and provide real-time integration. The Big Data and analytic result sets may need to be abstracted and delivered as reusable data services in order to allow different interaction models such as discover, search, browse, and query. These practices ensure a Big Data solution that is not only cost effective, but also one that is flexible for being leveraged across the enterprise.
Several technologies and approaches serve the Big Data needs of which two categories are particularly important. The first has received a lot of attention and involve distributed computing across standard hardware clusters or cloud resources, using open source technologies. Technologies that fall in this category and have all received a lot of attention include Hadoop, Amazon S3, Google Big Query, etc. The other is data virtualization, which has been less talked about until now, but is particularly important to address all three spokes in the wheel of (Big Data) life mentioned above:
– Data virtualization accelerates time to value in big data projects: Because data virtualization is not physical, it can rapidly expose internal and external data assets and allow business users and application developers to explore and combine information into prototype solutions that can demonstrate value and validate projects faster.
– Best of breed data virtualization solutions provide better and more efficient connectivity: Best of breed data virtualization solutions connect diverse data realms and sources ranging from legacy to relational to multi-dimensional to hierarchical to semantic to big data/NoSQL to semi-structured web all the way to fully unstructured content and indexes. These diverse sources are exposed as normalized views so they can be easily combined into semantic business entities and associated across entities as linked data.
– Virtualized data inherently provides lower costs and more flexibility: The output of data virtualization are data services which hides the complexity of underlying data and exposes business data entities through a variety of interfaces including RESTful linked data services, SOA web services, data widgets, or SQL views to applications and end users. This makes Big Data reusable, discoverable, searchable, browsable and queriable using a variety of visualization and reporting tools, and makes the data easily leveraged in real-time operational applications as well.
CIOs and Chief Data Officers alike would do well to keep the three spokes of wisdom in the Big Data wheel of life in mind. They will realize the truth that every Big Data project needs the Ying-and-Yang balance between the Big Data technologies for storage and processing on the one hand and data virtualization for data access and data services delivery on the other. By following this path, good Karma is sure to be bestowed on both themselves and the customers they serve. AUM.
Go to source
- Data Governance in a Data Mesh or Data Fabric Architecture - December 21, 2023
- Moving to the Cloud, or a Hybrid-Cloud Scenario: How can the Denodo Platform Help? - November 23, 2023
- Logical Data Management and Data Mesh - July 20, 2023