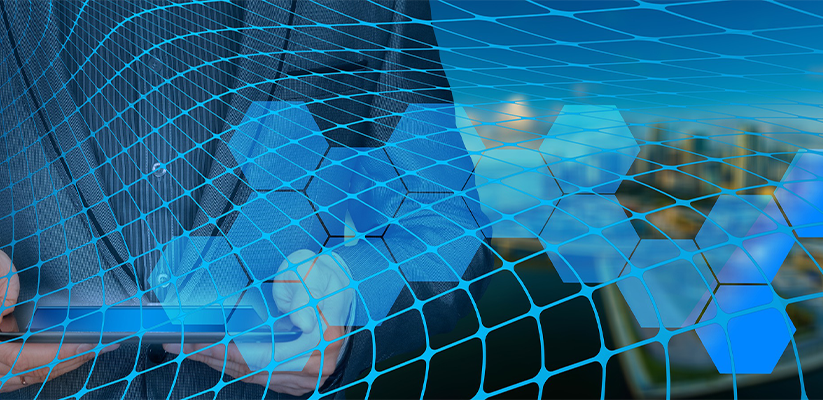
In this increasingly digital economy, we have seen major change in customer behavior over the last several years as customers switch from traditional channels to digital ones to interact and transact business. Today, the vast majority of purchases take place via mobile, social, and e-commerce applications as opposed to face-to-face. And if anything, the COVID-19 pandemic has accelerated the move to online as companies have been forced to go there to survive. The problem with all this is that if you want to create customer intelligent applications, you need to integrate customer data and insight and share it across all customer facing applications. However, many companies are struggling with this.
This impact of this is that customers are no longer interacting with employees to do business as used to be the case in traditional channels. Instead, they are interacting with applications over a relatively short period of time. Therefore, if companies want to keep customers loyal, improve customer engagement, and personalize each customer’s experience, then these customer facing applications need to become “customer intelligent.” If they don’t, the chances are that customers will quickly find alternative providers of products and services that more closely meet their needs and switch to those competitors in a few mouse clicks.
No Central Platform for this
In marketing for example, many are using multiple applications to market their products and services across multiple channels. Different applications may be used to control emails, digital banner advertising, or the placement of advertising words. Each of these applications has its own underlying database, which means marketing data is fractured across different systems with an unknown degree of overlap. There is no central customer master, and no single integrated view of all customer insights that can be easily shared across multiple digital marketing applications. In short, marketing departments often lack a central platform for delivering customer data, and this lack causes real problems.
For example, customer segmentation is being repeated in different channels using different databases for use in different marketing applications. In digital marketing, the data needed to determine return-on-investment is fractured across internal systems and marketing service providers which means the measurement of digital marketing effectiveness lacks detail. In short, fractured data is leading to marketing overspend. Also, fully weighted marketing attribution to optimize campaign spend by segment across each channel to maximize conversion rates remains an aspiration rather than a reality.
The same is true in sales, where again, different applications may be in use, each with their own database. Customers may be treated differently across channels because data is not integrated and shared. Also, personalization is often limited, e.g. based just on monitoring online clicks rather than detailed, integrated, historical customer purchasing and online behavior data. Fractured data also impacts customer service, where the ability to see a history of customer interactions across all customer communication channels is also lacking. The lack of integrated data and analytics also restricts the ability of customer service representatives to make sales during customer interactions.
Looking at these problems, it is clear that integrated customer data and analytics shared across all front-office functions and channel applications is critical for success. Also, more data is needed from internal and external sources to get a more comprehensive understanding of customers. This includes customer master data and data about their lifestyles and interests, as well as data about their purchases, opinions, relationships, interactions, and behaviors. Also, new customer analytical workloads are needed to produce new customer insights. Companies want to use analytics to convert voice interactions into text, to analyze opinion and score sentiment, to identify customer relationships and understand customer online behavior. All of these new analytical workloads may be happening on different analytical systems, but once the new insights are produced, they need to be integrated with the customer purchase history (typically found in a data warehouse) and customer master data to provide a much richer understanding of each and every customer, available in a single-customer view that can be shared across all front-office functions and customer facing applications.
Data Virtualization and Customer Data
Data virtualization makes this possible because it enables customer insights created in different underlying analytical data stores to be presented as if they were all in one database, using virtual views. This provides a 360-degree view of the customer for use by all front-office functions and applications across all channels, creating the foundation for a customer-intelligent front office.
Once this richer set of customer data has been made available in a single-customer view, machine learning models can then analyze this data to improve customer segmentation (more segments that are smaller in size) and personalization. More accurate customer segmentation enables more effective multi-channel marketing campaigns, each aimed at a smaller segment, which should yield better sales conversion rates. In addition, smaller customer segments also help provide better intelligence on customer buying patterns.
Finally, prescriptive analytics can be developed to analyze the rich set of integrated customer data provided by data virtualization to facilitate personalized customer recommendations such as next-best offer and dynamic pricing. These prescriptive models can then be deployed as services so that personalized customer recommendations can be served up to all customer facing front-office applications, regardless of whether the application is being used by a customer-facing employee or a partner, or by customers themselves.
- Enabling a Customer Data Platform Using Data Virtualization - August 26, 2021
- Window Shopping for “Business Ready” Data in an Enterprise Data Marketplace - June 6, 2019
- Using Data Virtualisation to Simplify Data Warehouse Migration to the Cloud - November 15, 2018