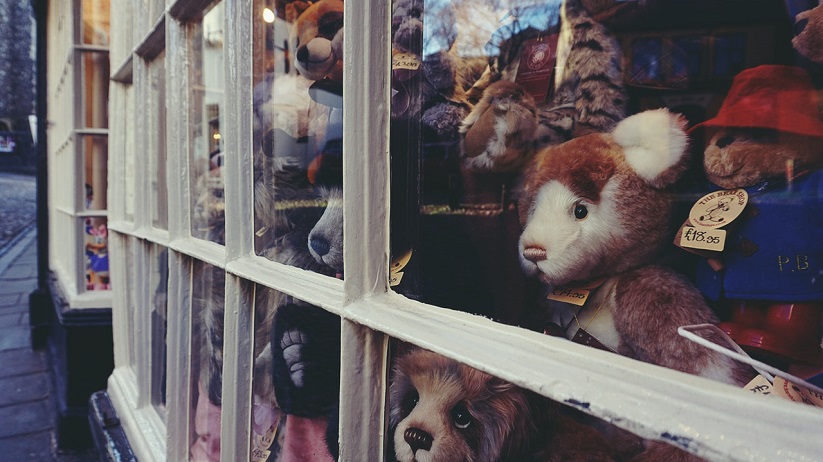
In many businesses today, data complexity is already a challenge with more and more data stores both on-premises and in the cloud as well as thousands of possible data sources. The days of just having a data warehouse and some data marts for query, reporting and analysis have long been surpassed by many additional analytical platforms optimised for specific analytical workloads such as streaming platforms, Hadoop, Spark as a service with data in cloud storage, graph databases, edge devices and analytics etc. All of this in addition to the one or more data warehouses you might have.
Why did the data complexity problem arise?
The reason this has come about is the demand for new data to analyse. This would include machine-generated data such as Clickstream data from web server logs as well as human generated data like tweets, customer inbound email and review web site data. All of this is pouring into the enterprise and needs to be cleaned and integrated to ready it for analysis.
The demand for self-service tools
Historically that task was given to IT. However, with all the new data being collected, business users often view IT as a bottleneck unable to keep pace with their needs. Therefore, it’s no surprise that they are demanding the ability to prepare data themselves using so called self-service data preparation tools that can either be bought separately or that come bundled with BI tools for example.
Self-service siloes
The result has been an explosion of personal data silos created by users with self-service data preparation tools are all preparing and integrating data from various sources and no-one sharing what they create. Also, there is a high risk that the same data is being inadvertently transformed again and again by different individual users. The result is that the cost of data preparation is way higher than it should be, re-use is minimal and inconsistently reigns.
So, the question is, if this individual self-service siloed approach is going on, do we just live with it or is there a way to remove the inconsistency, avoid chaos, remove that inconsistency and produce data assets that people can trust, and re-use across the enterprise? How do you eliminate re-invention, enable collaborative, agile development and reuse? Is there a better way? The answer is of course yes.
Window shopping for “business ready” data
It is to create an enterprise data marketplace which acts as an internal ‘shop window’ for ready-made data and analytical assets that people can pick up and use in ‘last-mile’ processing to deliver value. The way in which this can be achieved is by:
• Establishing a centralised (e.g. cloud storage, HDFS) or logical (an organised set of data stores) data lake within which to ingest raw data from internal and external data sources
• Creating an information supply chain whereby information producers can work together in project teams to:
- Curate this data to produce trusted, physical data products (assets) that can be stored in one or more trusted data stores
- Produce trusted analytics to process, score and cluster data
• Publishing trusted, curated data and analytical assets in an information catalog which acts as an enterprise data marketplace
• Simplifying access to those trusted data assets in one or more data stores by creating virtual data views that integrate this data using data virtualisation software
• Use data virtualisation to create new trusted virtual data views on top of trusted physical data assets and publish these as trusted virtual data assets to the data marketplace to build on what is stored physically
This is shown in Figure 1 below:
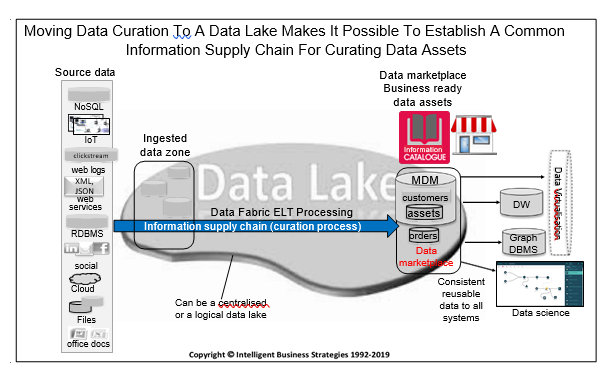
The idea behind an information supply chain is similar to a manufacturing production line. Its purpose is to act as a process for curating data products. That means from an organisational perspective, we have information producers who curate ‘business ready’ data products and information consumers who shop for this business ready data and use it to deliver value.
The whole point here is to produce trusted, commonly understood, ready-made data products that can be re-used in multiple places. The benefit is that we can save information consumers considerable amounts of time because they do not have to prepare all the data they may need from scratch. Instead we create ready-made data products or assets that are already fit for consumption. An example might be customer data, product data, orders data etc. Having data ‘ready-to-go’ should therefore shorten the time to value and reduce costs.
Figure 2 shows it in a different way:
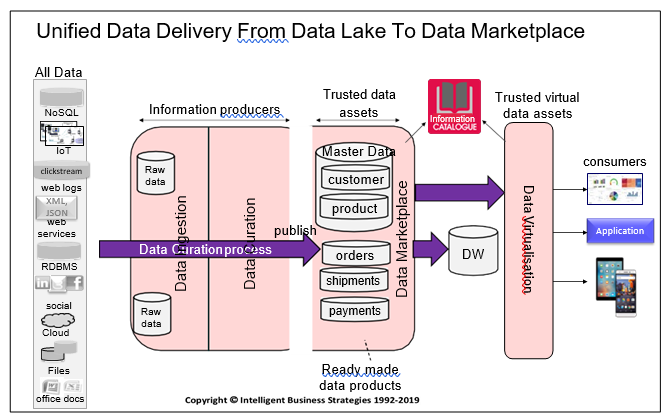
The objective is to produce ready-made data products and publishing them to enable business people to shop for data but without the need to distribute copies of this data.
The solution to making this possible while also giving you the flexibility to create new trusted data assets quickly is data virtualisation. In a world where data complexity is on the rise, companies have to put in place the foundations for a data-driven enterprise that enables business to quickly and easily find the data they need, know that they can trust it, and be able to access it quickly to deliver value. The role of data virtualisation integrated with a data catalog in making this possible is paramount.
- Enabling a Customer Data Platform Using Data Virtualization - August 26, 2021
- Window Shopping for “Business Ready” Data in an Enterprise Data Marketplace - June 6, 2019
- Using Data Virtualisation to Simplify Data Warehouse Migration to the Cloud - November 15, 2018